A key enabling technology for remote machine operation is the ability to estimate and control the full machine state. In the TracMac project we will devise and implement novel methods for estimating the state of articulated manipulators: using remote depth sensor measurements. We will verify the validity and utility of the state estimate by using it in a feedback controller. By removing all sensor hardware from the manipulator, we will greatly increase hardware robustness to damage and reduce repair and maintenance costs.
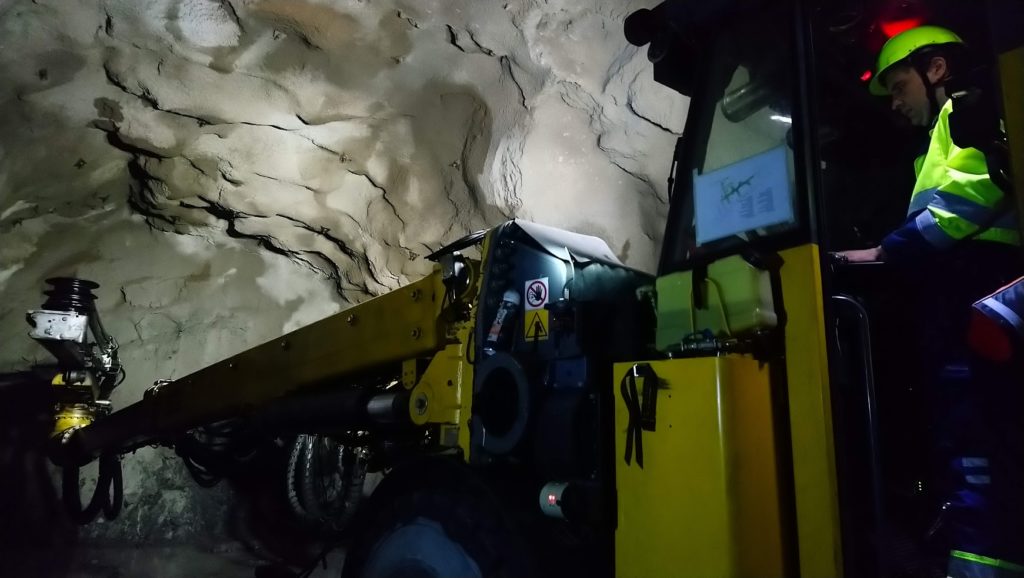
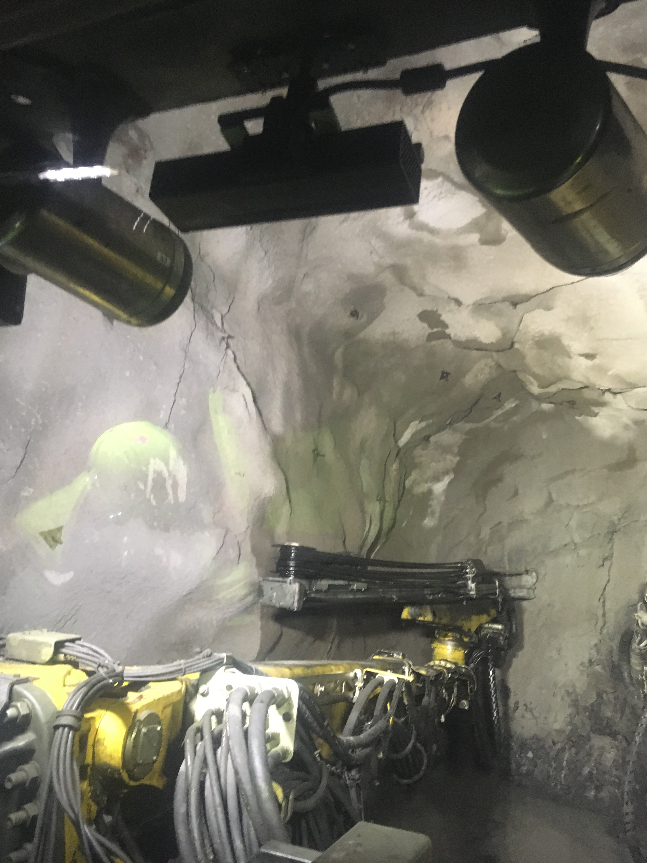
In TracMac we investigate visual learning architectures for recovering the full state of a partially visible manipulator. The problem is especially challenging, as the environment conditions are difficult to predict and model: noise patterns can vary substantially in between different mining tunnels.
TracMac is a 3 year project funded by Vinnova SIP STRIM. The project is coordinated by Orebro University and involves as partners Epiroc Drills AB, Zingruvan Mining AB, and the Alfred Nobel Science Park. For further details contact Todor Stoyanov.
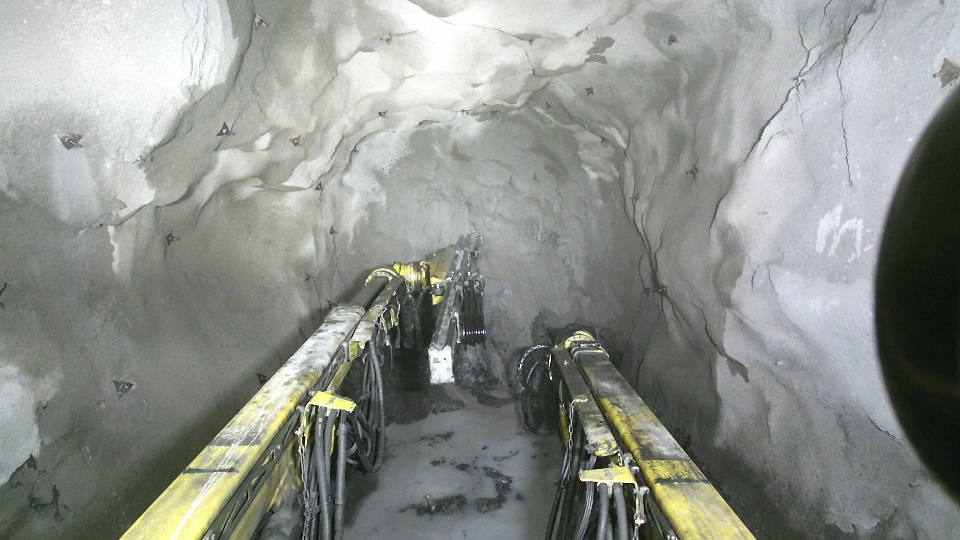
Recent News
Learning to Propagate Interaction Effects for Modeling Deformable Linear Objects Dynamics
Learning to Propagate Interaction Effects for Modeling Deformable Linear Objects Dynamics Abstract Modeling dynamics of deformable linear objects (DLOs), such as cables, hoses, sutures, and…
TracMac project at IROS 2019
Late breaking results from the TracMac project were presented at the International Conference on Robots and Systems (IROS) 2019 in Macau, China. We presented some…