The purpose of this project is to investigate the application of classical Artificial Intelligence (AI) techniques to the problem of Reinforcement Learning (RL) in continuous state/action spaces. This project is funded by the Wallenberg Autonomous Systems Program for Artificial Intelligence (WASP-AI) and is carried out in collaboration with partners at Lund University.
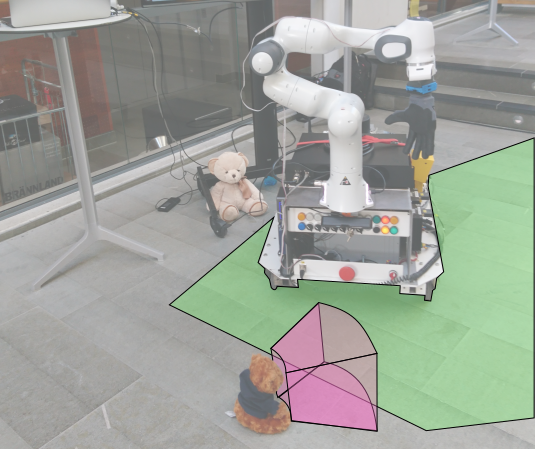
At the AMM Lab we are responsible for developing novel solutions to reinforcement learning that are suitable for deployment on real robots. We are investigating different methods for integrating prior knowledge and prior data sets collected for similar tasks or via demonstration.
The researchers involved in this project are Quantao Yang, Johannes A. Stork and Todor Stoyanov.
Recent papers
MPR-RL: Multi-Prior Regularized Reinforcement Learning for Knowledge Transfer
Abstract In manufacturing, assembly tasks have been a challenge for learning algorithms due to variant dynamics of different environments. Reinforcement learning (RL) is a promising…
Null space based efficient reinforcement learning with hierarchical safety constraints
Null space based efficient reinforcement learning with hierarchical safety constraints Abstract Reinforcement learning is inherently unsafe for use in physical systems, as learning by trial-and-error…